I am a postdoctoral researcher at the Data Science Institute (DSI) and Department of Brain Sciences, Imperial College London, specialising in generative AI for medical imaging. My research focuses on developing deep generative models for cardiac diagnostics, including digital twins for personalised healthcare.
I completed my DPhil at Fudan University, in a joint programme with Imperial College London, where my thesis on breast cancer diagnostics received top honours.
I have been awarded the Dame Julia Higgins Fund (as PI and Co-PI) and recently selected as a DAAD AInet Fellow for AI in Science.
My research interests include medical image analysis, generative models, and data augmentation. I have published over 30 papers in leading journals and conferences, such as MICCAI, IEEE TMI, and Nature Cardiovascular Research. You can find my publications on Google Scholar.
🔥 News
- 2025.03: Invited as a Visiting Researcher at Alan Turing Institute
- 2025.03: 🎉 One paper is accepted by Nature Machine Intelligence
- 2024.12: Awarded Imperial College Research Fellowship
- 2024.11: Selected as a speaker for TEDxRutlish
on December 19th, 2024.
- 2024.10: Selected as a DAAD AInet Fellow for the Postdoc-NeT-AI 11/2024 Virtual Networking Week on AI for Science
- 2024.10: Invitated talk at Fudan University
- 2024.10: Received funding as PI from the Dame Julia Higgins Fund
- 2024.10: Received funding as Co-PI from the Dame Julia Higgins Fund
- 2024.09: One paper is released at arxiv
- 2024.09: 🎉 One paper is accepted by Nature Cardiovascular Research
- 2022.10: I join BioMedIA
, Imperial College London as a research associate in UK!
📝 Publications
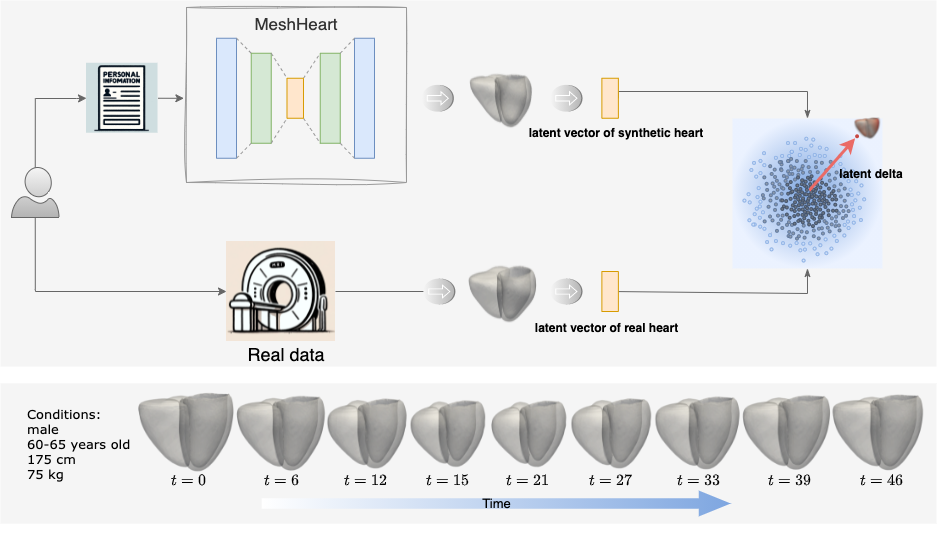
A Personalised 3D+ t Mesh Generative Model for Unveiling Normal Heart Dynamics
Mengyun Qiao, Kathryn A McGurk, Shuo Wang, Paul M. Matthews, Declan P O Regan, Wenjia Bai
- Developed a novel 4D mesh generative model for personalized cardiac anatomy reconstruction.
- Demonstrated improved accuracy in representing normal heart dynamics compared to baseline models.
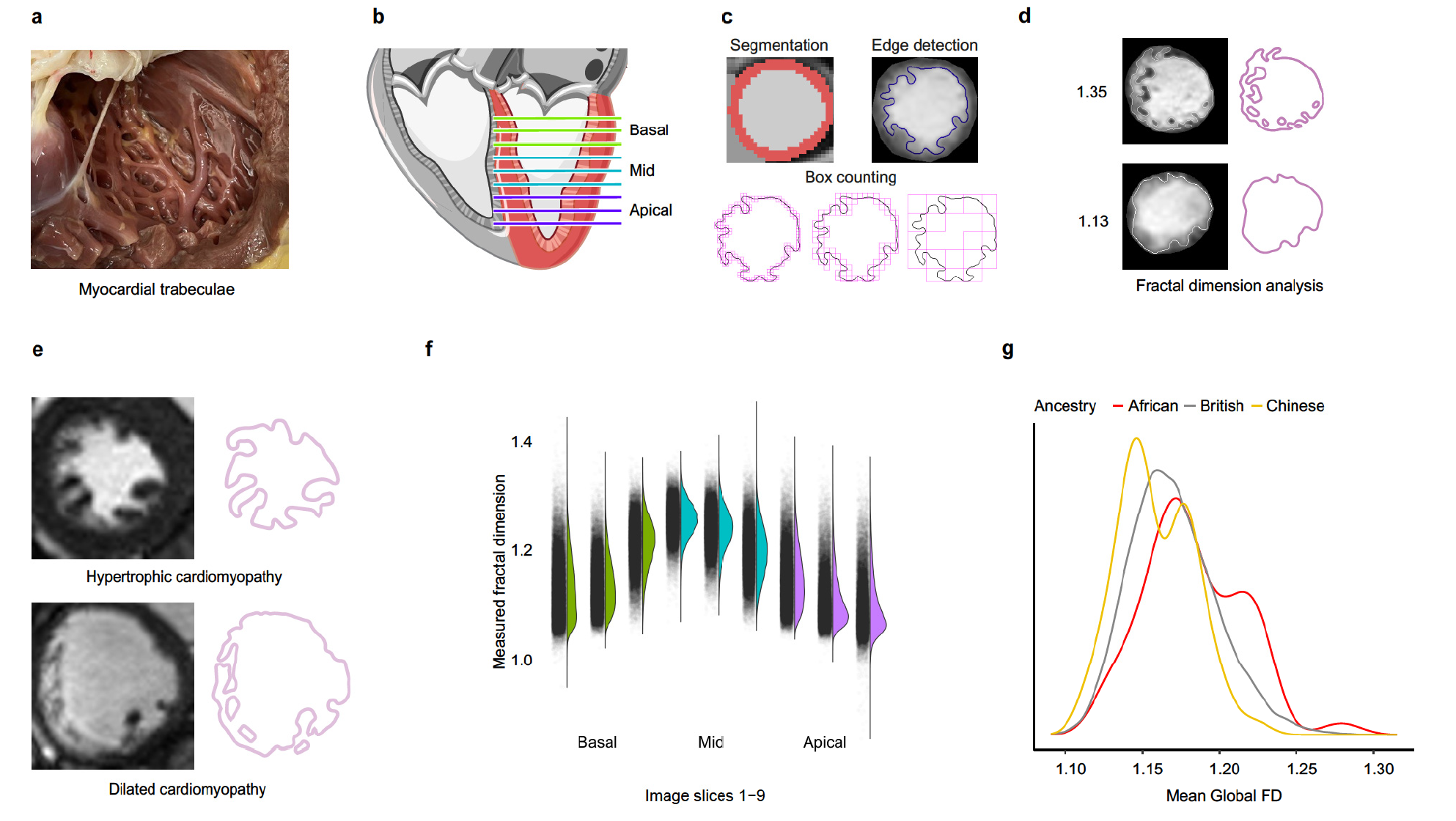
Genetic and Phenotypic Architecture of Human Myocardial Trabeculation
Kathryn A. McGurk, Mengyun Qiao, et al.
- Investigated genetic factors influencing myocardial trabeculation patterns.
- Identified novel phenotypic correlations linked to cardiac health indicators.
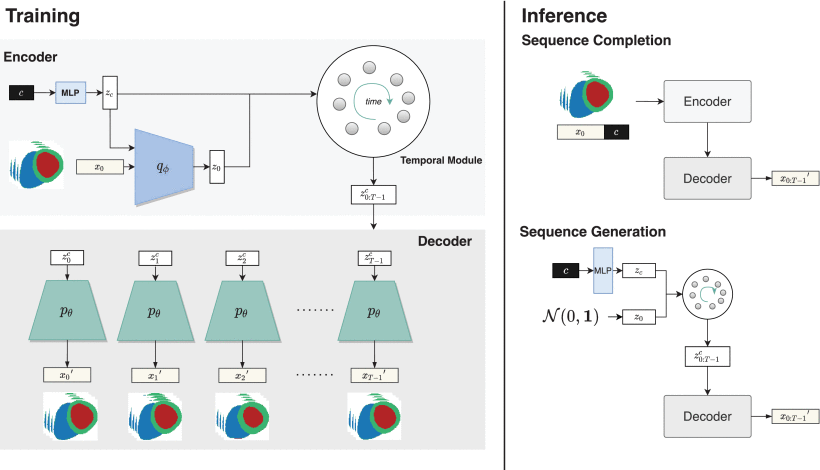
CHeart: A Conditional Spatio-Temporal Generative Model for Cardiac Anatomy
Mengyun Qiao, Shuo Wang, Huaqi Qiu, Antonio de Marvao, Declan P. O’Regan, Daniel Rueckert, Wenjia Bai
- Introduced a conditional generative model to capture spatio-temporal variations in cardiac anatomy.
- Achieved significant improvements in cardiac disease prediction over existing methods.
🎖 Fundings
- 2025-2029 Imperial College Research Fellowship (Principal Investigator).
- 2024-2025 Dame Julia Higgins Postdoc Collaborative Research Fund (Principal Investigator): Multi-Modality Digital Twin for Personalised Cardiac Diagnostics.
- 2024-2025 Dame Julia Higgins Postdoc Collaborative Research Fund (Co-Investigator): Disease Clusters in People with Metabolic-Dysfunction Associated Steatotic Disease and Multimorbidity.
- 2024 DeepMind UROP (Principal Investigator): AI-Heart Lifespan: Integrating Cardiac Imaging and Clinical Factors to Predict Heart Longevity.
- 2023-2024 Dame Julia Higgins Postdoc Collaborative Research Fund (Co-Investigator): Wearable Urine Volume Measuring Device for Bladder Function Monitoring.
📖 Education
-
2017.09 - 2022.06: DPhil in Biomedical Engineering, Fudan University, Shanghai, China
Awarded the best thesis of the year. Developed intelligent diagnostic algorithms for breast cancer using multimodal medical images. Published five peer-reviewed papers and presented at major conferences. -
2021.04 - 2022.06: Joint PhD Research, Imperial College London, BioMedIA Group, Department of Brain Sciences
Conducted collaborative research focusing on advanced machine learning and generative models for medical imaging. -
2017.06 - 2017.09: Visiting Researcher, Leiden University Medical Center, Department of Radiology, The Netherlands
Investigated cardiac motion tracking methods, achieving significant accuracy improvements using deep learning techniques. -
2013.09 - 2017.06: BEng in Electronic Engineering, Fudan University, Shanghai, China
Graduated with high distinction. Developed a breast ultrasound image analysis system to improve diagnostic efficiency. Awarded best undergraduate thesis of the year.
💬 Invited Talks
- 2024.10, London Symposium 2024, IDEA Lab, Friedrich-Alexander University Erlangen-Nuremberg: Personalised Generative Models for Unveiling Normal Heart Dynamics
- 2024.07, Shenzhen University: Deep Generative Modelling for Cardiac Anatomy
- 2023.09, 1st CCF Digital Medicine Symposium (DMS), Suzhou: Application and Optimization of Generative AI in Medical Multimodal Data
- 2020.05, IEEE ISCAS (Virtual Talk): Breast DCE-MRI Radiomics: A Robust Computer-Aided System Based on Reproducible BI-RADS Features
- 2019.07, IEEE EMBC, Berlin: Breast Calcification Detection Based on Radiofrequency Signals by Quantitative Ultrasound Multi-Parameter Fusion
- 2018.09, STACOM Workshop, MICCAI, Granada: Fully Automated Left Atrium Cavity Segmentation from 3D GE-MRI